Harnessing the Power of Labeled Image Datasets for AI Success
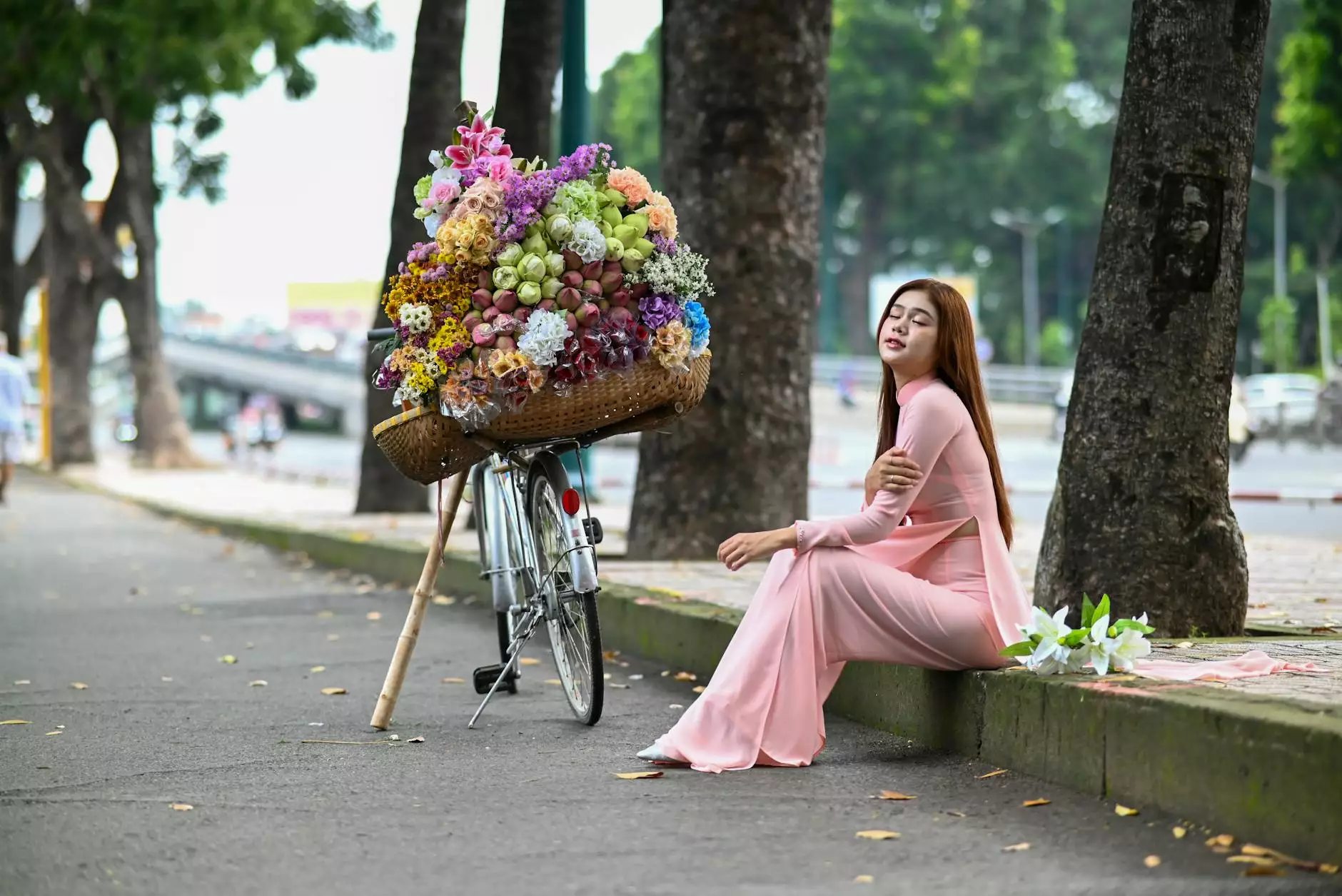
In today’s rapidly evolving technological landscape, labeled image datasets play a pivotal role in propelling advancements in artificial intelligence (AI) and machine learning. They serve as the fuel that drives neural networks and supports a myriad of applications from autonomous vehicles to facial recognition systems. Understanding the significance of these datasets and how to effectively utilize them can vastly enhance AI capabilities, setting businesses apart in a highly competitive market.
What are Labeled Image Datasets?
A labeled image dataset is a collection of images that have been tagged with specific annotations that provide contextual information about the content of each image. These labels can denote various attributes including, but not limited to, object presence, segmentation details, and classification categories. For instance, a marked image of a cat might include tags like "animal", "mammal", and "pet" within its associated metadata.
Types of Annotations
Annotations in labeled image datasets can be categorized into several types, each serving unique purposes:
- Bounding Boxes: These are rectangular frames around objects in images, often used for object detection tasks.
- Semantic Segmentation: This involves labeling each pixel in an image to classify parts of the image into categories.
- Instance Segmentation: Similar to semantic segmentation, but this method differentiates between distinct objects of the same class.
- Landmarks: Specific points on the images that identify key features, commonly used in facial recognition techniques.
The Importance of Labeled Image Datasets in Machine Learning
In machine learning, the effectiveness of algorithms significantly depends on the quality and quantity of labeled data available for training models. Here’s why labeled image datasets are so critical:
1. Enhancing Model Performance
Models trained on high-quality labeled image datasets exhibit better performance metrics during validation and testing. The reason is simple: they learn from accurately annotated real-world examples, enabling them to generalize better when exposed to unseen data.
2. Facilitating Fine-Tuning and Transfer Learning
Pre-trained models can be fine-tuned on specific domains using labeled image datasets. This transfer learning approach allows businesses to leverage established learning from one domain and adapt it to another, significantly reducing training time and computational costs.
3. Improving Operational Efficiency
Incorporating labeled image datasets allows for the automation of various tasks, such as image classification and detection, thereby improving operational efficiency. With machines capable of performing these tasks, human workers can focus on more complex and strategic activities.
Keylabs.ai: Your Partner in Data Annotation
When it comes to creating and managing labeled image datasets, partnering with a reliable data annotation service is vital. Consider what Keylabs.ai offers:
Comprehensive Data Annotation Tools
Keylabs.ai provides state-of-the-art data annotation tools that simplify the labeling process. These tools are designed to enhance the productivity of your annotation teams, ensuring consistency and accuracy in the creation of labeled datasets. The platform supports various annotation types, accommodating the needs of different projects, whether it's simple image tagging or complex segmentation.
Data Annotation Platform
The data annotation platform at Keylabs.ai boasts a user-friendly interface that empowers teams to annotate images effectively. With built-in quality assurance features, businesses can rest assured that their labeled datasets meet the highest standards, which is crucial for training effective machine learning models.
Best Practices for Creating Labeled Image Datasets
To derive maximum benefits from your labeled image datasets, consider the following best practices:
1. Define Clear Objectives
Before starting the labeling process, outline clear objectives of what you aim to achieve with your dataset. This could include the specific tasks for which you intend to train your model, such as detecting objects, recognizing faces, or segmenting images.
2. Use Diverse Image Sources
To ensure robustness in your model, source images from various contexts and environments. Diversity in data helps the model generalize better, making it capable of performing reliably across different scenarios.
3. Maintain High Annotation Quality
Invest in quality by employing skilled annotators or utilizing advanced tools that enforce annotation standards. Regular audits and feedback loops can further enhance the accuracy and reliability of your labeled image datasets.
4. Continuous Iteration and Update
The field of AI is constantly progressing. Continuously updating your labeled image datasets with new data and annotations ensures your models remain effective and aligned with current trends.
Future Trends in Labeled Image Datasets
The landscape for labeled image datasets is dynamic, reflecting the rapid changes within AI technology. Here are some trends to watch for:
1. Synthetic Data Generation
As AI technology advances, the generation of synthetic labeled images is becoming more prevalent. This method provides a cost-effective way to create diverse datasets needed for training, particularly when real-world data is scarce.
2. Increased Focus on Privacy and Ethics
With the growing concern for privacy, there is a stronger emphasis on creating labeled datasets that respect user data consent and adhere to ethical guidelines. Businesses will need to be proactive in ensuring their practices meet compliance requirements.
3. Automation in Data Annotation
Automation powered by AI and machine learning is likely to transform the data annotation process. By automating repetitive tasks, businesses can significantly speed up the creation of labeled datasets while minimizing errors.
Final Thoughts
The significance of labeled image datasets in the realm of artificial intelligence cannot be overstated. They are instrumental in driving advancements and enabling common applications we see in today’s digital world. By leveraging cutting-edge tools and platforms such as those offered by Keylabs.ai, businesses can ensure they have the right datasets to fuel their machine learning models and drive success.
As the industry evolves, staying updated with best practices and trends regarding labeled image datasets will be crucial for maintaining a competitive edge. Embrace the future of AI, where the quality of your data can significantly impact your outcomes, and take the necessary steps to curate top-notch labeled datasets.