Unlocking the Potential of Machine Learning Labeling Service
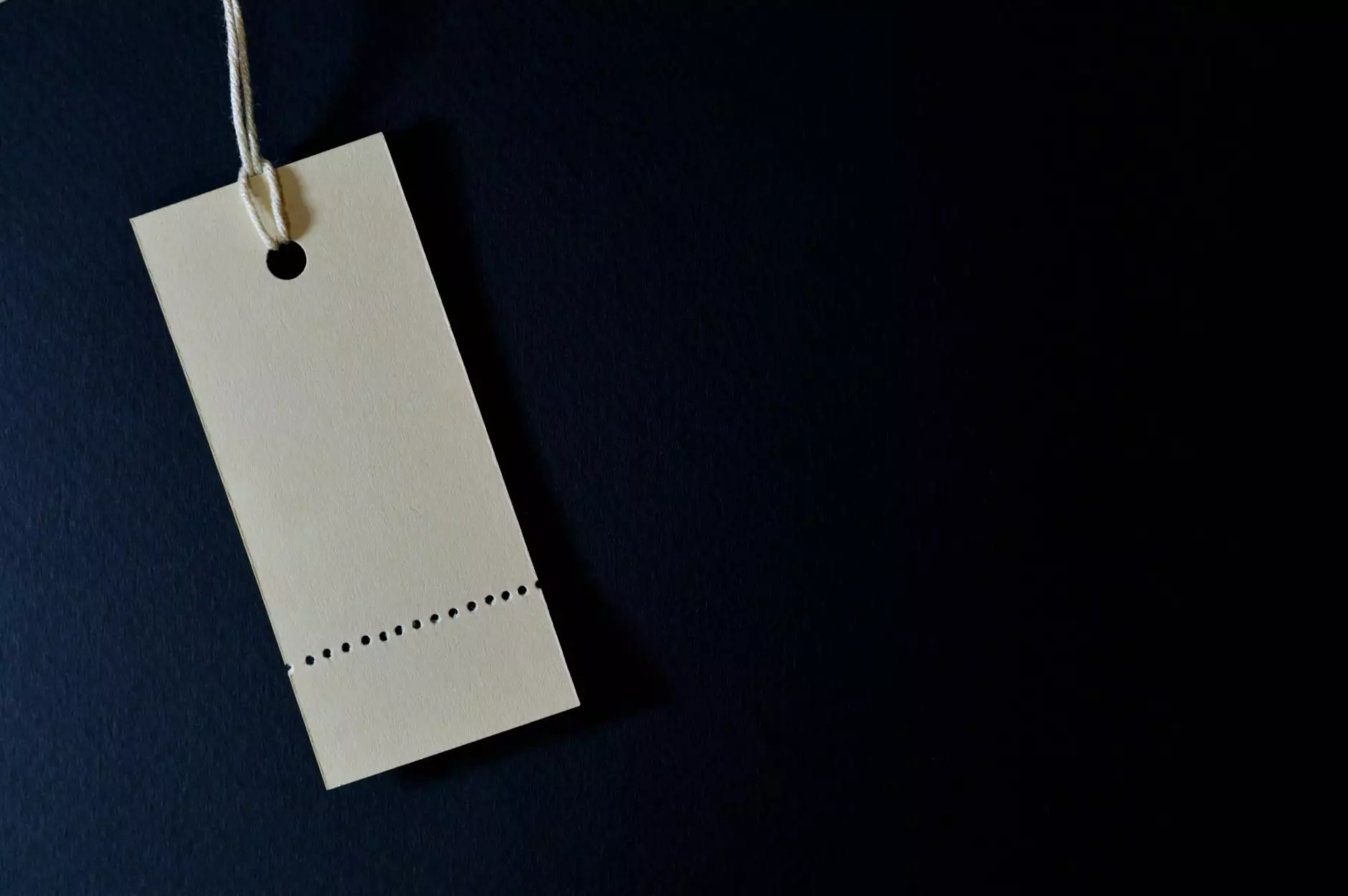
Machine learning labeling service plays a pivotal role in the landscape of artificial intelligence (AI). It serves as the foundational building block for training robust machine learning models. As businesses increasingly integrate AI solutions into their operations, understanding the intricacies of data labeling becomes crucial for achieving superior results in software development.
Understanding Machine Learning and Data Labeling
At its core, machine learning involves algorithms that learn from data, identify patterns, and make decisions. However, for these algorithms to function effectively, they require properly labeled data. This is where machine learning labeling service comes into play.
Why is Data Labeling Essential?
Data labeling converts raw data into a structured format that can be easily utilized by machine learning models. Properly labeled datasets assist algorithms in understanding the context and significance of data points. Here are some key reasons why data labeling is essential:
- Improved Model Accuracy: Labeled data provides the necessary context that helps algorithms make accurate predictions.
- Efficient Training Process: Machine learning models trained on high-quality labeled datasets perform better during testing phases.
- Wide Application: Data labeling enables a variety of applications, including image recognition, natural language processing, and predictive analytics.
The Process of Machine Learning Labeling Service
The labeling process typically involves several steps designed to ensure data quality. Understanding these steps can help businesses appreciate the value that machine learning labeling service offers:
Step 1: Data Collection
The first step involves gathering data relevant to the task at hand. This could include images, text, audio, or video files, depending on the application. The quality and diversity of data are crucial for building a reliable dataset.
Step 2: Choosing a Labeling Tool
Next, appropriate labeling tools are chosen based on the type of data and the complexity of the labels required. Various platforms offer customized tools suitable for specific industries.
Step 3: Annotation Guidelines
Creating clear and comprehensive annotation guidelines ensures consistency in labeling. This step is critical for minimizing ambiguity and maximizing the accuracy of labels.
Step 4: Human Labeling
In many instances, human annotators label data through manual processes or semi-automated tools. This step often demands attention to detail and subject matter expertise to accurately interpret data.
Step 5: Quality Assurance
After labeling, data must undergo quality checks to ensure accuracy. This can involve sampling labeled data for validation, employing automated checks, or second-pass reviews by additional annotators.
Step 6: Feedback and Iteration
Feedback mechanisms are integral for continual improvement. Annotators should have the opportunity to learn from mistakes and refine their understanding of labeling guidelines.
Types of Data Labeling Techniques
Different machine learning applications necessitate various labeling techniques. Some commonly used techniques include:
- Image Annotation: Techniques like bounding boxes, polygons, and semantic segmentation help in identifying objects within images.
- Text Annotation: Labeling of text data can range from sentiment analysis to named entity recognition, categorizing words or phrases based on their contextual meaning.
- Audio Annotation: Involves labeling audio segments to recognize specific sounds or speech patterns, crucial for voice recognition applications.
- Video Annotation: Combining image and time-based techniques, video annotation tracks movement and identifies patterns within video data.
Benefits of Utilizing Machine Learning Labeling Services
Embracing a professional machine learning labeling service comes with an array of benefits that can significantly enhance your AI projects:
1. Expertise and Experience
Professional labeling services employ qualified annotators skilled in various domains, ensuring high-quality output. Their experience aids in understanding nuanced data requirements which in-house teams may overlook.
2. Scalability
In a rapidly evolving business landscape, the ability to scale data labeling efforts is paramount. Professional services offer the capability to handle large volumes of data in a timely manner.
3. Cost-Efficiency
By outsourcing labeling to experts, businesses can save on costs related to training, tools, and labor while ensuring the quality of work remains high.
4. Focus on Core Business
Outsourcing allows organizations to concentrate on their core competencies while leaving data labeling to specialists. This enhances productivity and fosters innovation within organizations.
Choosing the Right Machine Learning Labeling Service Provider
With numerous players in the market, selecting the ideal machine learning labeling service provider can be daunting. Here are some factors to consider:
1. Quality Assurance Processes
Evaluate the provider's quality control measures. Look for companies that prioritize accurate results through thorough quality checks and feedback systems.
2. Technology Utilization
Assess whether the provider utilizes state-of-the-art technology to enhance the labeling process. AI-assisted tools can significantly boost efficiency and accuracy.
3. Flexibility and Customization
Every project requires specific approaches. Ensure the service provider is willing to tailor their solutions to meet your unique requirements.
4. Proven Track Record
Look for testimonials, case studies, and success stories to gauge the effectiveness of the provider’s services.
Future Trends in Machine Learning Labeling Services
As machine learning technologies evolve, so too will the practices surrounding data labeling. Several emerging trends include:
A. Automated Labeling
The advancement of AI and machine learning technologies will lead to increased automation in data labeling processes, reducing reliance on manual intervention and increasing efficiency.
B. Enhanced Collaboration Tools
As collaborative work environments gain traction, tools that foster collaboration between annotators and data scientists will enhance the labeling process.
C. Real-time Annotation
Future tools may enable real-time feedback and annotation, allowing for more dynamic labeling processes as data evolves or new patterns emerge.
Conclusion
The role of machine learning labeling service within software development cannot be understated. As the demand for AI applications continues to grow, leveraging high-quality labeled data will be imperative for crafting effective machine learning models. Organizations must recognize the importance of data labeling and consider partnering with expert service providers to maximize their AI initiatives.
Investing in a reliable machine learning labeling service is not merely a tactical decision but a strategic move to ensure long-term success in the evolving technological landscape.